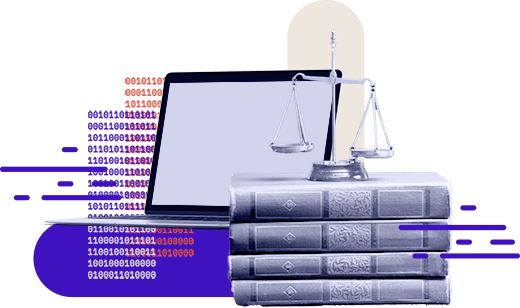
We use data science to find predictors for court decisions
Explore the pillars of our research:
Publications on legal research methods and data science
-
Schepers, I., Medvedeva, M., Bruijn, M., Wieling, M., & Vols, M. (2023). Predicting citations in Dutch case law with natural language processing. Artificial Intelligence and Law. Advance online publication. https://doi.org/10.1007/s10506-023-09368-5
- Medvedeva, M. (2022). Identification, Categorisation and Forecasting of Court Decisions. University of Groningen. Link: https://research.rug.nl/files/236807645/Complete_thesis.pdf
- Medvedeva, M., Wieling, M., & Vols, M. (2023). Rethinking the field of automatic prediction of court decisions. Artificial Intelligence and Law, 31(1), 195-212. https://doi.org/10.1007/s10506-021-09306-3
- Vols, M. (2021). Legal Research: One hundred questions and answers. Eleven International Publishing. https://www.
boomdenhaag.nl/webshop/legal- research. - Vols, M. (2021). Methodologische kansen en beperkingen van gebruik van statistiek en machine learning bij de bestudering van rechtspraak. In P. W. J. Verbruggen (Ed.), Methoden van systematische rechtspraakanalyse: Tussen juridische dogmatiek en data science (pp. 125-141). Boom Juridisch. https://research.
rug.nl/en/publications/ methodological-opportunities- and-limitations-of-using- statistics-. - Medvedeva, M., Üstün, A., Xu, X., Vols, M., & Wieling, M. (2021). Automatic judgement forecasting for pending applications of the European Court of Human Rights. In K. D. Ashley, K. Atkinson, L. K. Branting, E. Francesconi, M. Grabmair, V. R. Walker, B. Waltl, & A. Zachary Wyner (Eds.), Proceedings of the Fifth Workshop on Automatec Semantic Analysis of Information in Legal Text (ASAIL 2021) (pp. 12-23). CEUR Workshop Proceedings. https://pure.rug.
nl/ws/portalfiles/portal/ 189865814/Medvedeva_ASAIL2021_ 1_.pdf. - Medvedeva, M., Dam, T., Wieling, M., & Vols, M. (2021). Automatically identifying eviction cases and outcomes within case law of Dutch Courts of First Instance. In E. Schweighofer (Ed.), Legal Knowledge and Information Systems (pp. 13-22). (Frontiers in Artificial Intelligence and Applications; Vol. 346). IOS Press. https://ebooks.
iospress.nl/doi/10.3233/ FAIA210312. - Medvedeva, M., Xu, X., Wieling, M., & Vols, M. (2020). JURI SAYS: An Automatic Judgement Prediction System for the European Court of Human Rights. In S. Villata, J. Harašta, & P. Křemen (Eds.), Legal Knowledge and Information Systems: JURIX 2020: The Thirty-third Annual Conference, Brno, Czech Republic, December 9–11, 2020 (pp. 277-280). IOS Press.
- Medvedeva, M., Vols, M., & Wieling, M. (2020). Using machine learning to predict decisions of the European Court of Human Rights. Artificial Intelligence and Law, 28(2), 237-266. https://doi.org/10.1007/s10506-019-09255-y
- Vols, M. (2020), Juridisch onderzoek, The Hague: Boom Juridisch 2020.
- Bruijn, L. M., & Vols, M. (2020). Upperdogs versus Underdogs: Judicial Review of Administrative Drug-Related Closure Cases in the Netherlands. Recht der Werkelijkheid, 41(1), 25-49. https://www.bjutijdschriften.nl/tijdschrift/rechtderwerkelijkheid/2020/1/RdW_1380-6424_2020_041_001_004
- Bruijn, L. M., Vols, M., & Brouwer, J. G. (2018). Home closure as a weapon in the Dutch war on drugs: Does judicial review function as a safety net? International Journal of Drug Policy, 51, 137-147. https://doi.org/10.1016/j.drugpo.2017.08.003
- Vols, M. & Jacobs, J.P.A.M (2017), Juristen als rekenmeesters: over de kwantitatieve analyse van jurisprudentie, in P.A.J. van den Berg & G. Molier (Eds.), In dienst van het recht, Den Haag: Boom Juridische uitgevers.
- Vols, M., Tassenaar, P.G. & Jacobs, J.P.A.M. (2015), Dutch Courts and Housing Related Anti-social Behaviour. A first statistical analysis of legal protection against eviction, International Journal of Law in the Built Environment, 7, pp. 148-161.